Product Management Data & AI: what missions, what profiles, what particularities?
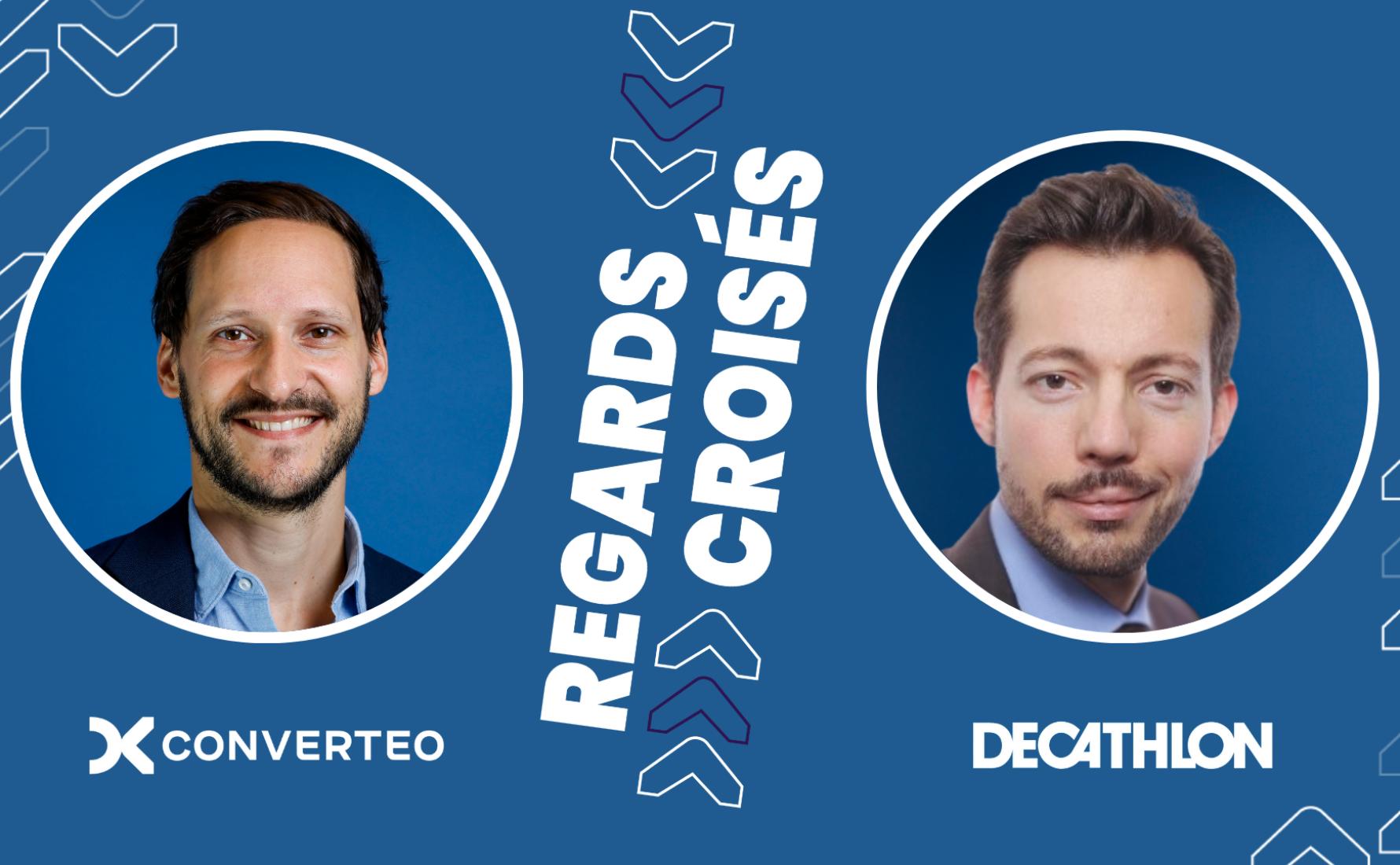
“Product managers” specifically dedicated to data and AI topics are starting to carve out a niche in organizations. But what are the particularities of these positions? How do they differ from traditional product managers? David Spire, Partner of the Product practice at Converteo, and Hervé Guignot, who leads the development of Data and AI Products at Decathlon, discussed the topic.
Positions related to Product Management for Data—and by extension AI—are still very recent in organizations…
David Spire: Indeed, the role of Data and/or AI Product Manager is primarily found in tech companies that are already quite mature in data issues and AI developments. In French companies, Product Management applied to data and AI is still a new concept. Some companies, like Decathlon, are pioneers in this area, but their example will likely be followed by many others soon.
Hervé Guignot: Yes, at Decathlon, we are fortunate to have a strong product culture, which stems from two factors. First, the company itself is not just a distributor: we design our own products, with brands like Quechua, Kalenji, or Nabaiji. Eighty percent of the products sold are developed internally. Second, over the past few years, we have completely transformed our approach to deliver the value of digital by building Product, Tech, and now Design teams that work together by domain. The Data teams naturally complement this setup, with the Data Product Manager constructing the data components of broader user experiences.
What are the essential skills for this role?
Hervé Guignot: I believe that three types of skills are essential: first, a deep and thorough knowledge of data and AI, understanding what can be done with them. Ideally, one should have “gotten their hands dirty,” meaning having experience as a data scientist or data analyst. The “product” dimension is also crucial: having a user-centered mindset, understanding the process (discovery, roadmapping, etc.), and the relevant tools. Finally, a certain level of “business” perspective seems indispensable. This can be achieved through specific expertise—such as in pricing, logistics, etc.—or through exposure to numerous use cases and business strategies. In practice, these three components—data, product, and business—are rarely found in equal measure in a single person. It’s more like climbing a mountain; there are often multiple paths to reach the summit!
If I reflect on my own career, I started in software before moving into Strategy Consulting, then into Data starting in 2012, and Data Science since 2015. I have only developed my understanding of the Product approach in recent years.
However, I use all of this almost every day to, for example, engage with marketing, pricing, and finance teams on their topics and pain points, to understand the potential of a particular algorithm, or to develop a transformation roadmap.
David Spire: I believe that profiles capable of taking on a PM or PO role for Data products must necessarily have a significant skill track in the field. Just as we value a Web Product Manager who understands the technical implications of various development frameworks (like React), it is essential for a product manager working on data to understand how a data lake functions, etc. For an AI Product Manager, this is even more critical because the quality of the available data must be fully mastered to envision the experiences that can be delivered. Beyond that, being an excellent Product Manager is crucial: one must have a high level of seniority, as these are complex products that require a particular intensity of work with stakeholders who may not always master the available solutions.
Is there a typical profile for this type of position?
Hervé Guignot: Not really! Often, these are very “tech” profiles that have sought to move into product management roles, but other data product managers come from business backgrounds, accustomed to interacting with “business” stakeholders. In fact, it all depends on what the organization expects from its Data Product Manager: some are very focused on “delivery,” while others are more oriented towards “discovery” or the definition of strategy.
David Spire: Either you are, as Hervé says, someone who worked in data during your early career, or you have been very involved in data-related topics from the business side, giving you a strong understanding of the issues that data or AI can solve. In any case, I believe a strong interest in both areas is necessary, along with a proactive attitude to stay constantly updated, as things evolve very quickly.
When discussing “Product” applied to Data, do we find all the classic dimensions of Product Management? Are there any particularities?
Hervé Guignot: In principle, we find the same modes of operation, starting with the “discovery” phase, which helps identify the value proposition to be delivered later. This is the main interest of the product approach: to imagine the value proposition with users, paying attention to what brings them value, iterating, and prototyping. This is really the key point that wasn’t necessarily present in data topics in the past.
However, one of the specifics is that in data product management, we work with a raw material. On a website, for example, the only raw material is user demand, which can be worked on and modeled. In data, in addition to user needs, we work with a raw material that often constrains us and imposes additional steps: it is essential that the data be available, of good quality, and useful for use cases.
In data and AI, some UX rules are also quite specific—such as issues of explainability, uncertainty, or “feedback loops”—which exist much less in digital products. This necessitates training and mobilizing expert profiles on these topics.
David Spire: We must not forget that regardless of the product, the goal is to deliver experiences. So even if working on a data product or developing AI solutions remains specific, it does not prevent the final outcome from delivering a significant experience that addresses a clear, identified, and measurable problem. In this sense, I genuinely believe that the entire classic dynamic of Product Management is present. However, it is necessary, especially regarding AI, to find ways to manage a much higher level of uncertainty: the responses of generative AI are almost always different. Thus, minimizing this risk becomes a significant particularity.
Do data and AI address the same issues or represent two distinct topics?
Hervé Guignot: Yes, rather, even if it’s not always evident. It’s primarily a question of definition. Data encompasses analytics and business intelligence, while AI refers to data science or machine learning. However, when we create an AI model, even if there isn’t really a user interface, we must integrate it into other tools, such as a dashboard, to use and visualize it. This brings us back to a BI topic. The reverse is also true: if we design an analytics tool to provide figures and insights to users, we will naturally seek to integrate a predictive indicator, hence AI. Thus, the two tend to blend somewhat, even though there are differences in methodology and UX. Moreover, the profiles working on AI, analytics, and BI topics are not the same.
David Spire: From experience, data products are most often internally focused products that serve the operational departments of a company, even though there are also data products that support “front” experiences (for example, in personalization). In contrast, when it comes to AI, we are talking more about contributions aimed at enhancing the front-end experience at all levels—whether it’s an e-commerce site or a CRM. It’s global. In this sense, data and AI are two distinct application domains, but they feed into each other since AI cannot function without data.
How do you see this function evolving in the coming years?
Hervé Guignot: We will all continue to mature in data and AI for several more years. Technologies are constantly evolving, as are the ways to combine them and the usages. Therefore, I believe the role of Data Product Manager is here to stay, as it is essential to have truly expert profiles in data to design and deploy these new value propositions. In the long run, however, I think we will move towards much stronger integration with more traditional Product and Tech teams. After all, we serve the same customer, so we might as well do it together.
David Spire: The roles of Product Manager and Product Owner dedicated to data and AI products will become increasingly essential at all levels of organizations. This is already the case for data PO profiles, and it will become even stronger regarding AI, as AI is integrating into all layers of the company. This revolution will be as profound as the one brought by agility, which has been spreading within organizations since the 2000s. In other words, all products will see their scope expand to include AI-enabled experiences, so everyone who has a product management role, directly or indirectly, should take an interest in it if they want to continue having an impact by 2030!