Verteego and Converteo: AI Supporting Decision-Making in Retail
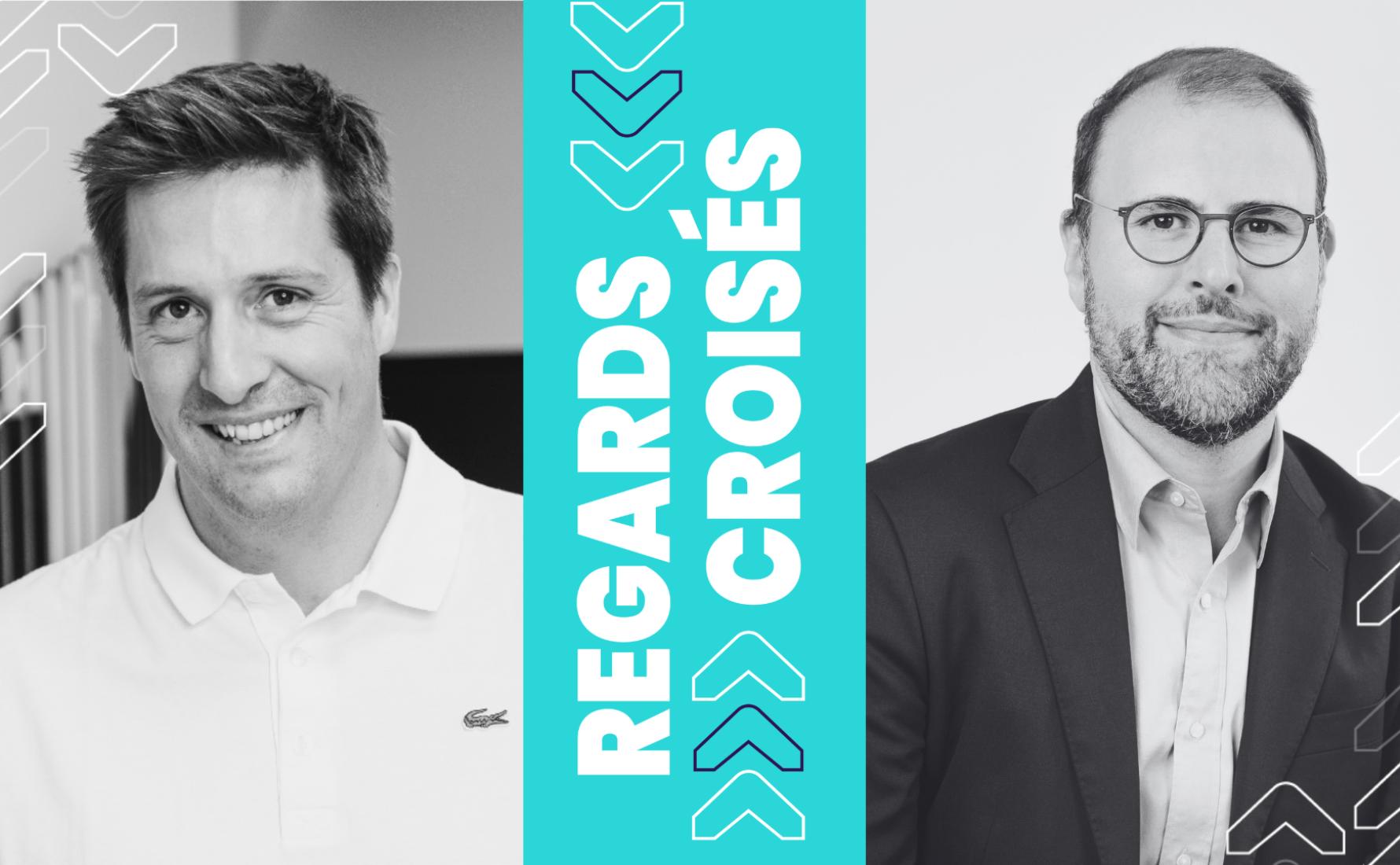
At the Tech for Retail conference—this year highlighting artificial intelligence—Rupert Schiessl, CEO and co-founder of AI solution provider Verteego, and Raphaël Fétique, senior partner and co-founder of Converteo, share their perspectives on the contributions of AI to decision-making.
-
To begin with, what is Verteego’s value proposition?
Rupert Schiessl: Verteego offers a platform within the AI intelligence ecosystem. It enables companies to make better decisions using data and technology, especially in e-commerce, large retail, or specialized retail. Rather than replacing existing tools, we complement clients’ ERP systems by analyzing real-time data and generating recommendations to optimize the supply chain, enhance marketing campaigns, or streamline logistics.
Raphaël Fétique: Verteego’s approach is highly complementary to Converteo’s. As software developers, Rupert and his team can advance deeply into R&D, while we provide field insights and client needs, facilitating the integration of their tools within companies. We have been discussing AI-related topics for a long time and have been increasingly strengthening our collaboration in recent years, including several joint projects—such as with Samsung, for example.
- Specifically, how does AI enable data to “speak” in order to facilitate decision-making?
Rupert Schiessl: There are three levels of sophistication in decision support tools. The first level involves using technology to process and analyze data to enable decision-makers to make informed choices.
The second level, known as “augmented decision making,” goes a step further: the algorithm provides recommendations that the user can choose to follow or not. In this case, it is particularly important to explain the origin of each recommendation, indicate which variables were used, or what the level of reliability of the forecasts is. The third level, “automated decision making,” allows the algorithm to make decisions autonomously in real-time.
Verteego operates primarily at the second and third levels.
Raphaël Fétique: For example, with Samsung, our goal is to optimize marketing expenditures for the e-commerce site by automating forecasts. To do this, ERP data alone is not enough. We need to feed the forecasting systems with other types of data, such as competitive environment data or media buying data. The challenge is to integrate these new data sources to enable more accurate forecasts. This is not straightforward, as the data sources are very diverse and not standardized.
One of our common goals with Verteego is to work on standardizing data sets to spend more time optimizing decision-making rather than preparing data.
-
How can new forms of generative AI help your clients address these various challenges?
Rupert Schiessl: With this new wave of generative AI, the question now is how to get users to effectively adopt these tools. We tell everyone they need to learn to master artificial intelligence, but what does that mean in practice? In this context, our responsibility towards users—whether technical or operational—is to support them in using these tools effectively. For example, ensuring they understand what constitutes a good recommendation and how it was developed.
Explainability of decisions is a major challenge in the development of our decision intelligence solution: it is a key parameter to facilitate adoption by teams. Generative AI can greatly improve this aspect by making reasoning and recommendations more readable and explicit for business functions.
Increasingly, our users want to have control and understand the process: we are working on this by evolving our interfaces and offering more natural language interactions.
Raphaël Fétique: Generative AI can also be very useful upstream, in the processing and cleaning of data, especially textual and semantic data. For example, internally, organizations rarely keep an up-to-date history of their promotional offers beyond a few years. In this case, scraping specialized promotional sites can help reconstruct this history.
Another example: on marketplaces, each supplier presents a product catalog with thousands or even hundreds of thousands of references. If these data are not standardized and corrected, it is difficult to perform meaningful analyses. Given the volumes involved, it has become impossible to perform this “cleaning” work manually.
Generative AI can thus play a key role in data curation and data qualification: it can be used to process large volumes of data and structure them. This is crucial because you cannot have a good forecasting model if the data used to feed it is not of high quality.