Data processing with AI: When weak signals become strong arguments
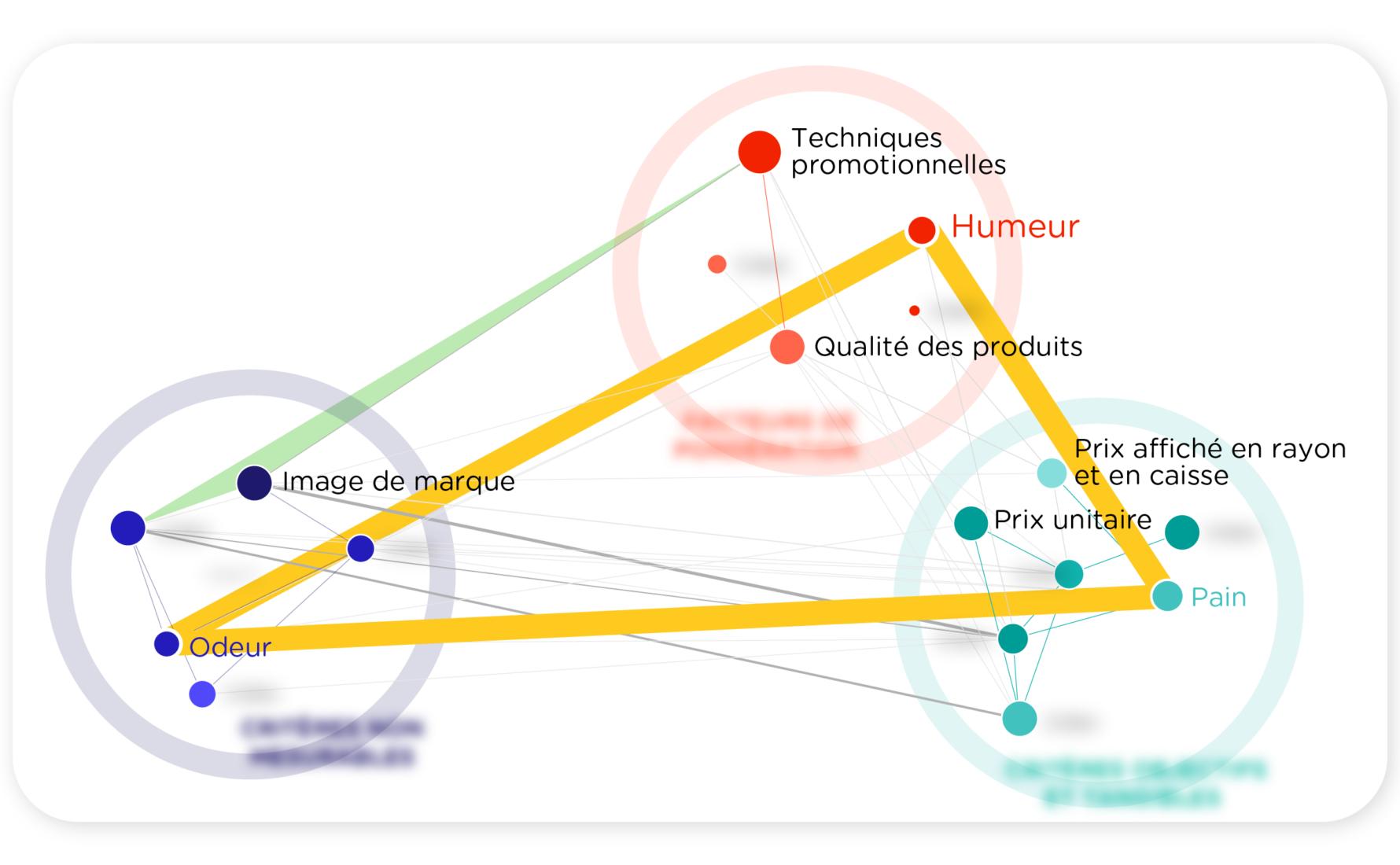
Context:
In a fictional letter to her grandmother, Natacha Dagneaud, founder of the qualitative research institute Séissmo, explains the benefits of artificial intelligence in text analysis and its use through concrete examples. This includes her collaboration with Synomia, a company specializing in artificial intelligence applied to semantics.
Hello Grandma,
You always say that I have a strange job. Yes, I observe people for hours, ask them questions (almost like a journalist!), and then interpret their answers—while paying close attention to their silences, their bursts of laughter… Because often, people don’t tell the whole truth. And that’s something you taught me!
You can imagine that during these long interviews, many different things are said. For example, we recently worked with 27 menopausal women, documenting their daily lives for seven days (through a blog, a sort of diary but on the internet). The result: 189 pages of responses to read, which amounts to precisely 92,669 words to analyze. Sure, counting words isn’t rocket science; Word does it very well—but be careful, that’s not artificial intelligence.
It’s true, you taught me to read very early (Do you remember Boris Vian’s novel “I Will Spit on Your Graves” just before I turned 15?). But reading and interpreting are not the same thing… Imagine that today, there are technologies that allow us to reinterpret our work.
Another example: we recently interviewed 40 people shopping in different supermarkets. These interviews were conducted using the “cognitive interview” method, which we ironically borrowed from the police.
The principle: for over an hour, the interviewees recount what they experienced in a specific recent situation (they are immersed in their memories as if they are reliving the scene). It’s a scientific way to enhance memory capacity and obtain more accurate testimonies. I’ll use it with you next time you’re looking for your keys 😊.
Anyway, we collected an average of 4,500 words per interview, which totals 180,000 words for the 40 people interviewed (excluding extraneous characters!).
How could my brain process such a volume? Obviously, I will filter a lot and highlight the aspects I am most aligned with. I know I need to be very careful, and my colleagues do too. Yes, we are not robots.
That’s why, a year ago, we reached out to numerous providers who could help us intelligently handle this data. It took us a while to find a quality partner, but we eventually found the company “Synomia” with whom we are collaborating on many projects. Synomia specializes in artificial intelligence applied to large-scale text analysis. Essentially, they identify and sort words in texts to help us uncover the ideas contained within them. They have their own technology and teams that support us in making the most of it.
They are based in Paris but can handle multiple languages in their software (we are currently using English, German, and French).
So far, they have worked with our quantitative colleagues, who deal with simpler sentences but in larger quantities. Now, they are also working with us, qualitative researchers, where the situation is reversed: we interview fewer people but produce more complex texts.
We collaborate closely with their R&D team to clarify our needs and requirements.
For example, the context from which each word was created is very important to us. They have therefore developed an additional feature that allows us to recontextualize a verbatim (i.e., the consumer’s original statement).
We have access to a dedicated platform where our texts—our interview subjects—are “stored” until we “theme” them, assigning them to a meaningful subject.
We can sort, search, group, and categorize topics and sensations. It’s about mastering chaos! But is it really that important to count words or quantify occurrences? After all, not everyone speaks the same way. And with this system, the talkative might end up being heard more, right?
However, when a topic doesn’t interest us, we tend to avoid discussing it! Similarly, we will warm up and talk extensively about a topic that touches us.
So in the end, it doesn’t matter what people think about a subject. What’s important is to identify whether the subject “generates buzz.” And as soon as they talk about it, we can derive meaning from it! That’s why we need to consider the “volume,” which is new for qualitative researchers.
I want to make you understand how much this software has changed my daily work (it’s a SaaS platform, similar to your subscription to Reader’s Digest, but virtual). It’s a bit like a washing machine. You need to carefully sort your laundry and set the right temperature. The machine doesn’t replace you or guess your intentions, but it performs the tedious task quickly and accurately if you control it properly. On the other hand, if you mix any raw materials together, you’ll end up with a mishmash.
It’s the same with artificial intelligence and syntax software (which simply means the computer understands the grammatical structure of sentences): clear analysis of verbs, adjectives, subordinate clauses, pronouns, phrases… It makes me smile because Latin lessons were not in vain. AI presents us with real challenges:
- On what principles do we sort this “laundry,” i.e., the texts? Can we include ALL consumer and moderator statements without overly distorting the overall meaning? The machine reveals that some interviewers talk a lot, sometimes too much.
- How do we transcribe audio recordings into electronic format? This takes time, costs money, and requires excellent training for transcribers.
- Another issue… What punctuation rules should we establish for transcribing oral conversations? The machine treats sentences as a unit and “cuts” them along punctuation marks. When someone describes an experience with hesitations, onomatopoeias, or speech tics (“and … mmmh then … finally …”), does this constitute a single sentence? Or should our transcribers use periods or semicolons, thus creating separate sentences? The total number of verbatims remains necessary to weight the topics. And this detail is far from insignificant.
- How do we make our clients understand that the interest also lies in the weight of words, and not just in the number of people talking about them? I actually wrote an article on this topic if you’re interested!
TO SEE THE ARTICLE ON THE WEIGHT OF WORDS, IT’S ALSO HERE
Thanks to this industrial-level processing, words are recognized, classified, and counted. Until now, we did it manually 😉: we identified significant reading units, wrote them down, and then compiled them into reports usually consisting of lengthy sentences. Until now, counting words or phrases was something no one would have thought of. It’s simply impossible by hand. In fact, our work hasn’t fundamentally changed: it’s still our analytical and interpretative skills that make the difference. But Synomia’s machine opens new possibilities for processing our raw material and allows qualitative researchers to deepen their investigations. For example, I like to focus on verbs during analysis because they reveal intention.
La somme des mots rend visible des phénomènes difficilement quantifiables à l’œil nu, comme le montre le graphique ci-dessous. Les clients qui ont fait leurs courses dans le magasin D ont visité moins de rayons et ont consommé un panier moyen plus petit.
Cette enseigne a donc du mal à séduire ses clients tout au long de leur parcours en magasin.
Figure 1: Number of Simple Sequences per Purchase Act
Finally, I have to tell you about some amusing details I discovered thanks to AI that I had never noticed before.
- First, the importance of bread: it was the eighteenth most frequently used word in our recent study on grocery shopping. I was of course aware that in a French-speaking country, when shopping, people almost always buy bread. But I never imagined that people would talk so much about bread as an important part of their in-store experience. It was when I cross-referenced this information with the sensory impressions consumers experience in a store that I had the revelation. Without the smell of bread, there is no joy in the store!
- Another time, I was surprised to discover the value of negation. I tend to focus more on positive affirmations: what a person does, chooses, handles… But the software is so intelligent that it also detects when people “do not do” something. It identifies the number of negations in a sentence. I was therefore able to determine in which stores shoppers exhibited avoidance behavior. In the most expensive supermarkets, consumers seemed to have internalized a sort of self-restraint; they emphasized in their accounts of their experiences that they had “not bought / not chosen” certain products.
- One last anecdote: during a study on anti-dandruff shampoo, which came in either a bright orange formula or a creamy white formula, the manufacturer wanted to know if the orange color was problematic. Instead of directly asking people and forcing them to give an answer, which would have biased their responses, we let them share their experiences with shampoo. We immediately noticed that the color scheme was a key element of the product’s identity, with strong associations.
That’s it, Grandma, there’s still much more to say, and I promise to keep you updated – but even in this letter, words are counted.