Strategy and technology: the two inseparable sides of pricing projects
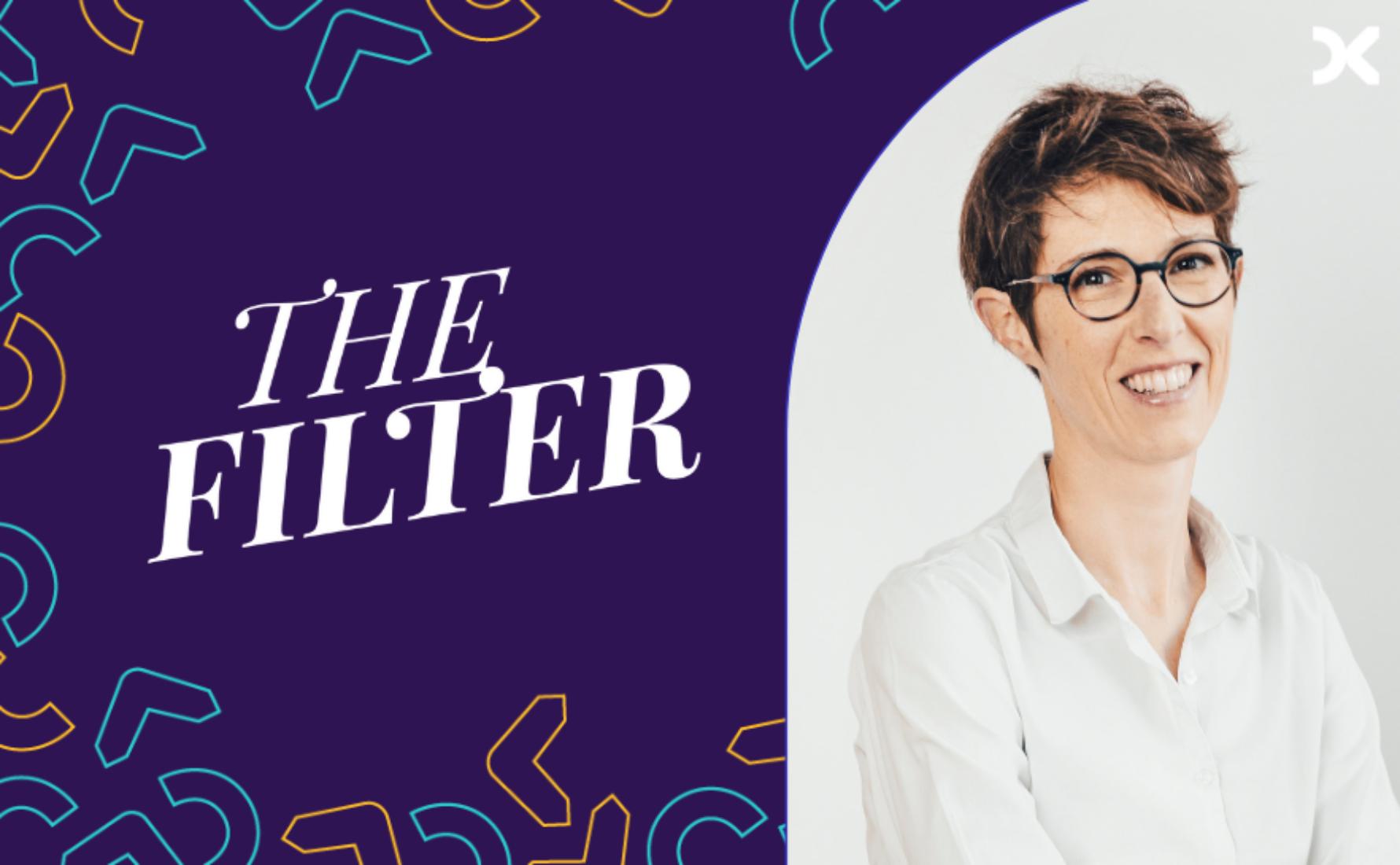
The Insight from… Emilie Gariel, Partner Data Business Consulting and Pricing Expert
With over ten years of expertise in pricing—first as a client-side professional at Rexel, then as a consultant at McKinsey, and later in SaaS software with the creation of Brennus Analytics—Emilie Gariel is Converteo’s leading authority on the subject. After sharing the convictions that led her to launch the Pricing Strategy, Operations & Solutions offering, she examines the transformative changes in pricing over the past decade.
Key Takeaways
- Over the past decade, the tools available to pricing teams have significantly advanced, enabling more flexible, responsive, and effective “data-driven” pricing strategies compared to the rule-based approaches of the past.
- Simultaneously, business teams have gradually gained skills and maturity, recognizing the value of data in their daily operations and decision-making processes. Pricing is increasingly seen (and managed) not as a “data maintenance” activity but as a strategic lever for margin protection and support for commercial strategy.
- However, it is crucial not to rely solely on technology for pricing strategy. Its full potential is only realized within a well-thought-out approach that considers the commercial, human, and organizational challenges unique to each company.
In the current economic context, there are numerous questions related to pricing strategies. How can one anticipate changes in logistics costs or raw materials and apply them at the right time, whether increasing or decreasing prices? How can one maintain volumes and market share in an uncertain environment? And, most importantly, how can one protect margins without overstepping?
These are crucial questions that proper use of data can help address. However, when it comes to setting prices, many organizations still rely on a quick competitive analysis or some basic market rules. While relying on intuition might have been sufficient a few years ago, it is no longer the case today.
Pricing has been profoundly enhanced by data
In the world of 2023, it is almost absurd to attempt to deploy a pricing strategy without considering the maximum amount of available data, both internal and external. Although the economic environment is more complex than in the past, commercial teams can now rely on increasingly powerful technologies to set and adjust their prices.
Ten years ago, data exploitation possibilities were limited due to the volume of available data and processing capabilities. Today, there are numerous exploitable data sources and efficient tools. Technology now allows for price optimization by considering a multitude of parameters, with a flexible and adaptable approach, almost in real-time.
The End of Hyper-Simplified Pricing
Although data has been incorporated into pricing strategies for a long time, its use has often been limited by available tools. Choices had to be made quickly to address two constraints: the ability to process data in Excel and the need for processes that were clearly understandable by teams. The result? Hyper-simplification of pricing, at the expense of optimization.
This situation explains a certain reluctance towards data, which can still persist today. However, technologies available on the market now allow for faster, better, and easier data processing than just a few years ago. Models, which were rudimentary in the 2010s, are now much more advanced, thanks to constraint optimization algorithms. The result is a much finer and more dynamic pricing strategy.
In this context, as data handling improves and becomes more effective, sticking to Excel often means missing out on a significant operational efficiency lever.
Teams Have Also Gained Maturity
In parallel, teams have gained maturity on these topics, particularly in commercial functions. Sales forces are becoming increasingly comfortable with data. As they see the value of data in their daily operations and decision-making, employees have progressively enhanced their skills in this area.
Confidence in the reliability of data has also increased, as the quality of available data has improved. Moreover, “black box” systems – which were the norm when AI began to democratize – are gradually being replaced by more transparency and clarity.
In the most mature organizations, data scientists now work hand in hand with business teams, considering the need for algorithm “explainability.” Operational teams can rely on performance analysis modules, optimization tool parameter settings, or result explanations.
Unfortunately, most organizations are not yet at this stage. Depending on their level of advancement, they use technology primarily to understand and analyze performance, identify anomalies, and find possible improvements (Step 1); establish business rules (Step 2); create “what if” scenarios by integrating models such as elasticity (Step 3). The most advanced organizations move on to full optimization of their pricing strategy (Step 4).
However, it is crucial not to fall into a common pitfall: assuming that data and tools do everything without considering the strategic, human, and governance issues surrounding their deployment. These aspects are specific to each case and organization.